

Dreblow and Associates
Dreblow and Associates
Prospecting is 100% of our Focus
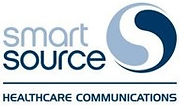
"Demystifying A.l. in the Healthcare Revenue Cycle"
Michael Laidlaw, Healthcare Revenue Cycle Consultant to SmartSource, LLC
Dinesh Gambler, PhD, President, First Outcomes.
Every day someone is touting "something new" with aftificial intelligence and how it is either going to save or destroy the world. ln the meantime, we believe it would be helpful to understand a little bit more about what they are talking about and how these applications apply to healthcare revenue cycle activities.
Al are "intelligent" program applications that can work independently or in concert with other programs to produce desired results.
The four basic "intelligent" applications are:
Speech Al
Al Vision (OCR)
Robotics Process Automation (RPA)
Before our apocalyptic demise, if you believe it or not, we will define each application, what it does and how it may be used in the revenue cycle. There are a number of companies out there that have developed some good and some not so good Al applications.
We believe having the knowledge of the applications and how they work within the revenue cycle will be helpful when you are making decisions that impact your department and your healthcare provider.
As you read through some of the examples, they may sound repetitive in that they are used multiple times; however, you will note that they may use a different AI application. For example, where you may use Speech Al for
Patient intake and registration you may also use AI Vision during the registration to translate paper documents to data that can be uploaded to your EHR.
There are multiple examples so it would be beneficial to have the right Al "tools" enhance all of your revenue cycle activities.
So let us begin:
Speech Al, also known as Speech Artificial Intelligence, refers to technologies that utilize artificial intelligence to enable machines to understand, generate, and interact using human language. lt involves two primary fields of study: Natural Language Processing (NLP) and Automatic Speech Recognition (ASR).
Natural Language Processing (NLP): This branch of Al is focused on the interaction between computers and human language. lt involves understanding, interpreting, generating, and manipulating human language in a way that is both meaningful and contextually appropriate. This is what allows Al to understand commands, questions, or even write and generatehuman-!ike text.
Automatic Speech Recognition (ASR)' This involves the conversion of spoken language into text. This technology is often used in applications such as voice assistants, transcription services, and any other application where spoken language needs to be understood by a computer.
These technologies, when combined, enable a variety of applications, including but not limited to virtual assistants (!ike Siri, Alexa, and Google Assistant), speechtotext transcription services, automated customer service systems, and many more. ln all of these, the aim is for the Al to understand and respond to human speech'as naturally and accurately as possible.
Here are examples of Speech Al in the healthcare revenue cycle
Patient lntake and Registration: Al can be used to streamline the process of collecting information from new patients or verifying information from existing ones. Speech recognition technology can transcribe spoken data into digital forms, which can reduce administrative workloads and minimize
errors. This typically takes the form of an incoming telephone call from the patient interacting with a digital employee who can assist with the registration.
Insurance Verification: Speech Al can interact with patients to gather their insurance information. The AI can understand complex insurance jargon and ask the necessary questions to clarify coverage details, thus ensuring the hospital or clinic has all the necessary information to bill correctly. This most often happens over the phone, outgoing call, when an eligibility check fails.

Appointment Scheduling and Reminders: Al can schedule appointments by communicating with patients via phone or text platforms. The Al can also remind patients of upcoming appointments, payment due dates, or necessary actions they need to take before an appointment. This can improve adherence and decrease the number of missed appointments, thus positively impacting the revenue cycle.
Billinq and Payment Processing: Al can explain medical bills to patients, address their questions, and process payments. Patients can answer questions regarding their bills or payment status, and the Al, with its ability to understand and respond to natural language, can provide relevant and helpful responses. This is mostly incoming calls where the patient wants to pay a bill.
Debt Collection: Speech AI can also be used in the collection process. For patients with outstanding balances, the Al can make reminder calls, explain the owed amount, and provide options for payment plans. It's able to do this in a manner that's respectful and understanding of the patient's situation, thus maintaining patient satisfaction while still promoting payment of debts. Research has found that only one in 7 calls are answered. AI, with its ability to call on a continuous basis, is critical in contacting patients and ensuring payment to the healthcare facility.
AIVISION , orArtificial lntelligence Vision Optical Character Recognition, refers to the application of Al technologies, specifically machine learning and computer vision, in the field of Optical Character Recognition (OCR).
Traditional OCR is a technology that is used to convert different types of documents, such as scanned paper documents, PDF files, or images captured by a digital camera, into editable and searchable data. However, it often struggles with recognizing text in complex layouts or poor-quality images.
AI-enhanced OCR solutions learn from the mistakes and the successes in recognizing text. They use machine Iearning algorithms to improve their ability to recognize text in a variety of fonts, colors, layouts, and even on different surfaces. lt can handle noisy backgrounds, poor lighting conditions, distortions, and other complexities that traditional OCR might struggle with.
Furthermore, by combining Al OCR with Natural Language Processing (NLP), these solutions can not only extract text but also understand the context and make sense of the information, just like a human would. This is extremely beneficial in fields Iike data entry, document analysis, invoice processing, and many others where manual text extraction could be time- consuming and error-prone.
Revenue Cycle Examples of Al OCR
Patient Registration and Data Entry: When patients fill out paper-based forms or submit digital forms with images or PDFs, Al OCR can be used to extract the data and populate it into the Electronic Health Record (EHR) system. This can save time and reduce errors associated with manual data entry.
Insurance Verification: Al OCR can scan and process insurance cards and other related documents. lt can extract information Iike policy numbers, coverage details, and patient identifiers to verify insurance and ensure accurate billing.

Coding and Billing: Al Vision can be used to scan and analyze clinical documents, extracting diagnostic codes, procedure codes, and other relevant information for accurate billing. This can also help in identifying any potential issues or discrepancies in coding or billing.
Claim Processinq: Al Vision working conjunction with NLP can be used to extract information from claim forms for faster and more accurate claim processing. It can analyze the forms to verify that all required information is included and accurately input into the system.
Pavment Posting and Reconciliation: When healthcare organizations receive payment details from patients or insurance companies (often in the form of checks, EOBs (explanation of benefits), or ERAs (electronic remittance advice)), Al Vision can extract the necessary details. This helps in the posting of payments to the correct patient accounts, reconciliation ofpayments, and the identification of any discrepancies or outstanding amounts.
Decision Trees and Robotic Process Automation (RPA) work together to enable automated decision-making in business processes.
Decision Trees: A Decision Tree is a type of predictive modeling tool. It uses a tree-like model of decisions and their possible consequences, including chance event outcomes, resource costs, and utility. lt is one way to display an algorithm that only contains conditional control statements. !n the context of automation, decision trees can be used to map out different outcomes based on a series of decisions, making it a useful tool for scenario analysis.
Robotic Process Automation (RPA): RPA is a technology that uses software robots (or'bots') to automate repetitive, rules-based tasks usually performed by humans. These tasks can include data entry, transaction processing, and responding to simple customer service queries.
When used together, decision trees can provide the decision-making framework that guides the actions of the RPA bots. For instance, an RPA bot might be programmed to take different actions based on the outcomes in a decision tree.
Some healthcare revenue cycle examples of combined decision trees and
RPA:

Patient Eliqibility Verification: Decision trees can be used to define the sequence of steps to verify a patient's insurance eligibility, while RPA bots can execute these steps. The bot would gather patient insurance details, login into the insurer website and validate them, and then update the patient's profile in the Electronic Health Record (EHR) system.
Appointment Scheduling: Decision trees can be used to guide patients through the process of scheduling an appointment based on factors such as their symptoms, the urgency of their condition, and the availability of doctors. RPA bots in conjunction with Speech Al can then automate the actual scheduling process in the EHR system based on the inputs gathered. The interaction with the patient and the review of the EHR scheduling system is done in real time using RPA.
Claims Submission and Follow-up: RPA bots can automate the process of submitting insurance claims and following up on their status. A decision tree can be used to determine the necessary steps based on the response from the insurance company (for example, if the claim is denied, the decision tree may guide the bot to resubmit with additional information).
Payment Postino and Reconciliation: Once payments are received from either the patient or the insurance company, RPA can automate the posting of these payments to the appropriate patient accounts in the system. Decision trees can help to determine how to handle discrepancies between billed and received amounts.
As William Shakespeare most eloquently stated in "The Tempest" "O, brave newworld that has such people in't!" We believe we are part of the "brave new world" and that artificial intelligence is a new frontier that will be of great value to everyone.
We hope that our explanations, examples and the application of Al in the healthcare revenue cycle have been helpful. Please contact us if you would like to know more about our Al journey and the "rise" of true "digital employees".
Thank you.
Timothy Moore
Director of Healthcare, Western Region
O: (800) 232-5004, ext. 353 | C: 805.551.4036
16634 Millikan Avenue | Irvine, CA 92691
tmoore@smartsourcellc.com
Matt Leventhal
Regional Sales Manager, West Coast Region
O: (800) 232-5004, ext. 261 | C. 949.293.4832
16634 Millikan Avenue | Irvine, CA 92691
MLeventhal@smartsourcellc.com
www.smartsourcelllc.com